Calendario
Estamos preparando nuevas convocatorias, déjanos tus datos a través del formulario y te avisaremos en cuanto estén disponibles.
Acerca del curso
En el curso ML Pipelines on Google Cloud aprenderás sobre TensorFlow Extended (TFX), que es la plataforma de machine learning de producción de Google basada en TensorFlow para la gestión de pipelines y metadatos de ML. Los primeros módulos analizan los componentes de pipeline, la orquestación de pipeline con TFX, cómo puede automatizarse el pipeline a través de CI/CD y cómo administrar los metadatos de ML. Luego, se analiza cómo automatizar y reutilizar pipelines de ML en múltiples marcos de ML, como tensorflow, pytorch, scikit learn y xgboost. También, aprenderás a usar Cloud Composer para orquestar tus pipelines de capacitación continua y MLflow para administrar el ciclo de vida completo del machine learning.
- Científicos de datos que busquen generar un impacto comercial mediante la conversión rápida del prototipo de machine learning a la producción.
- Ingenieros de software que busquen desarrollar habilidades de ingeniería de machine learning.
- Ingenieros de machine learning que deseen adoptar Google Cloud.
- Organizar el entrenamiento y la implementación de modelos con TFX y Cloud AI Platform.
- Operar modelos de machine learning implementados de manera efectiva y eficiente.
- Realizar una capacitación continua con varios marcos (Scikit Learn, XGBoost, PyTorch) y organizar pipelines con Cloud Composer y MLFlow.
- Integre los flujos de trabajo de machine learning con los flujos de trabajo de gestión de datos ascendentes y descendentes para mantener la gestión integral de linaje y metadatos.
- Haber completado el curso Machine Learning on Google Cloud.
- Haber completado el curso MLOps (Machine Learning Operations) Fundamentals.
Módulo 1: Introduction to TFX
- Develop a high level understanding of TFX standard pipeline components.
- Learn how to use a TFX Interactive Context for prototype development of TFX pipelines.
- Work with the Tensorflow Data Validation (TFDV) library to check and analyze input data.
- Utilize the Tensorflow Transform (TFT) library for scalable data preprocessing and feature transformations.
- Use the KerasTuner library for model hyperparameter tuning.
- Employ the Tensorflow Model Analysis (TFMA) library for model evaluation.
Módulo 2: Pipeline orchestration with TFX
Use the TFX CLI and Kubeflow UI to build and deploy TFX pipelines to a hosted AI Platform Pipelines instance on Google Cloud.
- Deploy a TensorFlow model trained using AI Platform Training to AI Platform Prediction.
- Perform advanced distributed hyperparameter tuning using CloudTuner and Cloud AI Platform Vizier.
Módulo 3: Custom components and CI/CD for TFX pipelines
Develop a CI/CD workflow with Cloud Build to build and deploy a TFX Pipeline.
- Integrate Github trigger to trigger Cloud Build CI/CD workflow for a TFX pipeline.
Módulo 4: ML Metadata with TFX
Access and analyze pipeline artifacts in ML Metadata store.
Módulo 5: Continuous Training with multiple SDKs, KubeFlow & AI Platform Pipelines
Perform continuous training with Scikit-learn and AI Platform Pipelines
- Perform continuous training with PyTorch and AI Platform Pipelines
- Perform continuous training with XGBoost and AI Platform Pipelines
- Perform continuous training with TensorFlow and AI Platform Pipelines
Módulo 6: Continuous Training with Cloud Composer
Perform continuous training with Cloud Composer
Módulo 7: ML Pipelines with MLflow
Manage Machine Learning lifecycle with MLflow
Módulo 8: Summary
Summarize the course
Documentación oficial para el curso ML Pipelines on Google Cloud.
- Formador certificado por Google Cloud.
- Más de 5 años de experiencia profesional.
- Más de 4 años de experiencia docente.
- Profesional activo en empresas del sector IT.
Solicita información
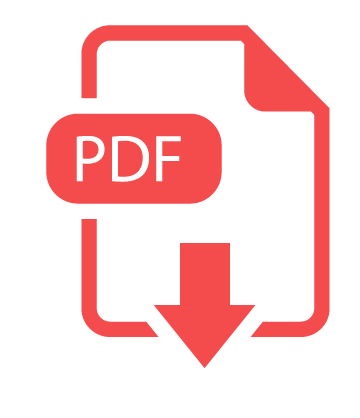
Descargar programa
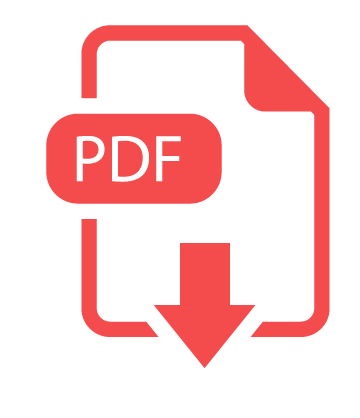
Descargar matrícula
Si no has encontrado lo que buscabas, prueba buscar tu curso o certificación aquí
Acerca del curso
El curso Advanced Machine Learning with TensorFlow on Google te brindará experiencia práctica en la optimización, implementación y escalado de una variedad de modelos de ML de producción. Aprenderás a crear sistemas de recomendación y modelos escalables, precisos y listos para producción para datos estructurados, datos de imágenes, series temporales y texto en lenguaje natural.
- Ingenieros de datos y programadores interesados en aprender a poner en práctica el machine learning.
- Cualquier persona interesada en aprender a construir y poner en funcionamiento modelos de TensorFlow.
- Implementar los diversos tipos de sistemas de producción de ML: capacitación estática, dinámica y continua, inferencia estática y dinámica, y procesamiento por lotes y en línea.
- Resolver un problema de ML mediante la creación de una canalización integral, desde la exploración de datos, el preprocesamiento, la ingeniería de características, la creación de modelos, el ajuste de hiperparámetros, la implementación y el servicio.
- Desarrollar una variedad de modelos de clasificación de imágenes, desde modelos lineales simples hasta redes neuronales convolucionales (CNNs) de alto rendimiento con normalización por lotes, aumento y transferencia de aprendizaje.
- Pronosticar valores de series temporales utilizando CNN, redes neuronales recurrentes (RNNs) y LSTMs.
- Aplicar ML al texto en lenguaje natural utilizando CNNs, RNNs, LSTMs, incrustaciones de palabras reutilizables y modelos generativos de codificador y decodificador.
- Implementar modelos de recomendación en TensorFlow, basados en contenido, colaborativos, híbridos y neuronales.
- Tener conocimientos de machine learning y TensorFlow al nivel de especialización Machine Learning on Google Cloud.
- Tener experiencia en codificación en Python.
- Tener conocimiento de estadísticas básicas.
- Tener conocimientos de SQL y computación en la nube.
Módulo 1: End-to-End Machine Learning with TensorFlow on GCP
Temas:
In the first course of this specialization, we recap what was covered in the Machine Learning on Google Cloud Specialization. One of the best ways to review something is to work with the concepts and technologies that you have learned. So, this course is run like a workshop where you will carry out end-toend machine learning with TensorFlow on Google Cloud Platform. Here you will learn how to explore large datasets for features, create training and evaluation datasets, build models with the Estimator API in TensorFlow, train at scale and deploy those models into production with Google Cloud Platform machine learning tools.
New learners with ML background can also follow this course to learn how to do ML on GCP to fast track to the more advanced topics coming soon under the advanced specialization.
Módulo 2: Production ML Systems
Temas:
We’ll cover how to implement the various flavors of production ML systems—static, dynamic, and continuous training; static and dynamic inference; and batch and online processing. We’ll delve into TensorFlow abstraction levels and the various options for doing distributed training and how to write distributed training models with custom estimators.
- Compare static vs. dynamic training and inference
- Manage model dependencies
- Set up distributed training for fault tolerance, replication, and more
- Export models for portability
Módulo 3: Image Classification Models
Temas:
We will take a look at different strategies for building an image classifier using convolutional neural networks. We’ll improve the model’s accuracy with augmentation, feature extraction, and fine-tuning hyperparameters while avoiding overfitting our data.
- Classify images using deep learning
- Implement convolutional neural networks
- Improve the model by augmentation, batch normalization, etc.
- Leverage transfer learning
Objetivos:
Gain an overview of how ML is applied to image classification, including the evolving methods and challenges
Módulo 4: Sequence Models
Temas:
- Predict future values of a time-series
- Classify free form text
- Address time-series and text problems with recurrent neural networks
- Choose between RNNs/LSTMs and simpler models
- Train and reuse word embeddings in text problems
Objetivos:
This module is an introduction to sequence models and their applications, including an overview of sequence model architectures and how to handle inputs of variable length.
Módulo 5: Recommendation Models
Temas:
- Devise a content-based recommendation engine
- Implement a collaborative filtering recommendation engine
- Build a hybrid recommendation engine with user and content embeddings
Objetivos:
Apply your knowledge of classification models and embeddings to build a ML pipeline that functions as a recommendation engine.
Documentación oficial para el curso Advanced Machine Learning with TensorFlow on Google.
- Formador certificado por Google Cloud.
- Más de 5 años de experiencia profesional.
- Más de 4 años de experiencia docente.
- Profesional activo en empresas del sector IT.